
Low Carbon Cities: creating a road map for retrofitting the Leeds social housing stock
Our model helps organisations decide on the best retrofit strategy to improve the quality of their housing stock.
Project overview
Domestic energy use accounts for a quarter of all greenhouse gas emissions produced by the UK. In order to meet the 2050 net zero target, retrofitting the existing housing stock is a priority. This is a complex and costly undertaking, and there are few guidelines, especially when it comes to large scale implementation. We created a tool to help organisations, such as Leeds City Council, decide on the best retrofit strategy for their housing stock and available resources.
Data and methods
We created a generic model to predict the cost and impact of retrofitting a given housing stock across an extended time period (default 2020-2050). The model is an Excel spreadsheet including VBA code, enabling easy and widespread use by non-experts.
Input data are derived from housing stock characteristics and possible retrofit activities. These can be adjusted easily to tailor the model to the local implementation. A set of parameters can be varied to examine the impact of the available budget, predicted future energy prices and emission factors, and behavioural effects related to residents and builders. This allows users to compare the outcome of different scenarios.
Three levels of prediction are available: at the level of individual houses, the level of eight house/flat archetypes (such as detached and semi-detached) and the ‘average’ UK house. The model optimises CO2 (equivalent) emissions saved each year, within budget constraints. Output includes yearly and cumulative costs and savings, including energy, emissions and energy bill savings.
Key findings
The ability to customise the model for the client organisation, such as tailoring input and output, and usability by non-expert users were important requirements.
In addition to the choice of input data, two other factors were found to have a strong impact on predicted energy savings, and hence emission savings.
First, the house-level model predicted energy savings that were only 50-65% of the savings predicted by the archetype level model. Without a gold standard (real energy usage e.g. from meter readings), it is difficult to tell which model offers a more accurate prediction.
Second, a model which only considers building physics (as used in most models) may significantly overestimate energy savings. Behavioural effects of residents (e.g. turning up the thermostat when their energy bill goes down) and builders (e.g. poor quality installation) are likely to explain a significant portion of the frequently observed mismatch between predicted and observed energy usage.
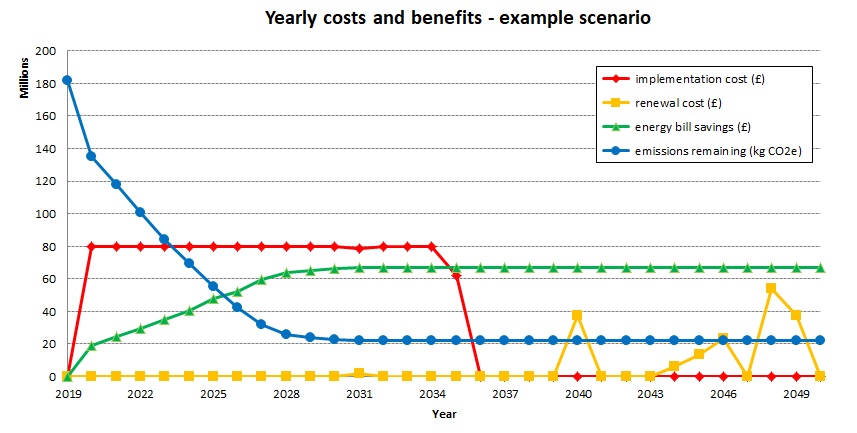

Value of the research
This model can be used by any organisation interested in exploring different retrofit strategies for their housing stock, such as councils and social housing providers. The model provides a quick and easy way to explore the potential costs and benefits of a variety of different scenarios.
Insights
- Making a model customisable and easy to use can promote adoption
- It is important to compare and adjust model predictions to the gold standard of real (metered) energy usage
- The possible impact of behavioural factors may often be underestimated
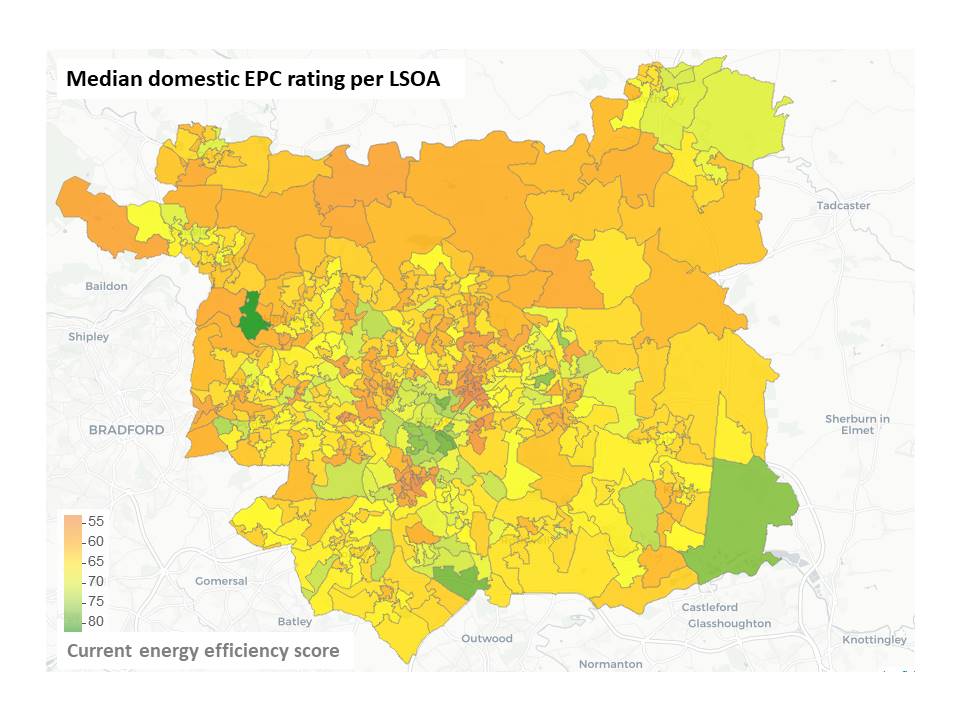
Research theme
Urban Analytics
People
Natalie Nelissen, Data Science Intern, Leeds Institute for Data Analytics
Andy Gouldson, Professor of Environmental Policy, School of Earth and Environment
Nick Malleson, Professor of Spatial Science, School of Geography
Alison Heppenstall, Professor in Geocomputation, School of Geography
Partners & Funders
Leeds City Council